Dr Shubham Chatterjee
Research Associate
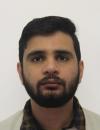
- Generalized Representation and Information Learning (GRILL) Lab
- Institute for Language, Cognition and Computation
- School of Informatics
Contact details
- Email: shubham.chatterjee@ed.ac.uk
Background
I am a Research Associate working with Dr. Jeff Dalton in the Glasgow Representation and Information Learning (GRILL) Lab, a leading research group in the School of Informatics at the University of Edinburgh that works on Conversational Information Retrieval. I am part of the Institute for Language, Cognition, and Computation. My research is in Information Retrieval, with an emphasis on Neural Entity-Oriented Information Retrieval and Extraction. The goal of my research is to develop novel algorithms that integrate information from text and entities present in the text to help search engines understand the meaning of the text more precisely. To this end, I heavily use tools and techniques from Natural Language Processing and Machine Learning, especially Deep Learning, in my work.
Prior to this, I worked as a Postdoctoral Research fellow with Dr. Laura Dietz at the University of New Hampshire, Durham, USA. This was also where I completed my PhD working with Dr. Dietz.
Research Interests: Entity-Oriented Search, Text Understanding, Neural IR, Conversational IR, Knowledge Graphs for IR, and Representation Learning for IR.
CV

Qualifications
PhD Computer Science. University of New Hampshire, Durham, USA. 2022.
MS Computer Science. University of New Hampshire, Durham, USA. 2020.
MSc Computer Science. University of Calcutta, Kolkata, India. 2017.
BSc (Hons) Computer Science. University of Calcutta, Kolkata, India. 2015.
Research summary
Large Language Models (LLMs) like ChatGPT have revolutionized our approach to language comprehension and generation. Yet, they face issues like hallucination -- generating information that lacks grounding in factual sources. To mitigate this, the integration of information retrieval mechanisms has emerged as a pivotal solution. These mechanisms allow LLMs to ground their responses in real-world, verifiable data, ensuring heightened accuracy and reliability. Thus, Retrieval Augmented Generation (RAG) has assumed paramount importance in modern AI applications, underscoring the critical need for the development of effective information retrieval systems. Entities, serving as explicit anchors in text, representing specific people, places, concepts, events, and more, emerge as indispensable assets in this context. While entities have historically proven their merit in feature-based information retrieval (IR) systems, modern neural IR models have scarcely tapped into their potential. As such, my research delves into advancing the utilization of entities within the AI ecosystem, with a particular focus on their role in bolstering the capabilities of information retrieval systems. My PhD work pioneered the integration of Knowledge Graph semantics into neural IR, diving deep into the interplay between entity semantics and neural IR's vector representations. For details, see my personal webpage.
Knowledge exchange
- I am currently co-organizing the TREC Interactive Knowledge Assistance Track
- I co-organized tutorials on Neuro-Symbolic Information Retrieval at SIGIR 2022 and ECIR 2022.