Ian Yang (AMRSC)
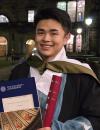
PhD Biological Sciences
Year of study: 1
- Swain Lab, School of Biological Sciences
- Grima Group, School of Biological Sciences
Contact details
- Email: ian.yang@ed.ac.uk
PhD supervisors:
Address
- Street
-
C.H. Waddington Building
Max Born Crescent
King's Buildings - City
- Edinburgh
- Post code
- EH9 3BF
Background
After his MChem and MSc degrees at the University of Edinburgh, Ian Yang discovered his passion for Systems Biology and was awarded a full scholarship from the Engineering & Physical Sciences Research Council (EPSRC) to study the metabolic oscillations of bakers' yeast using machine learning and mathematical modelling.
Qualifications
- 2024- : PhD Biological Sciences, University of Edinburgh
- 2023-2024: MSc Bioinformatics, University of Edinburgh
- 2018-2023: MChem Medicinal and Biological Chemistry, University of Edinburgh
Research summary
Characterising and understanding metabolic oscillations in single cells
Biological rhythms are a fundamental property of life, and even single cells can generate both cell cycles and circadian oscillations. My research aims at characterising and mathematically modelling metabolic oscillations in cells of bakers' yeast. My project uses a combination of machine learning, time-series analysis, and stochastic modelling as well as time-lapse microscopy of cells growing in microfluidic flow chambers under different nutrient conditions.
Current research interests
Machine Learning, Biophysics, Systems Biology, Mathematical Biology, Synthetic Biology.Past research interests
Computer-Aided Drug Design, Diffusion Models for Molecular Generation, Molecular Docking, Process Chemistry for Active Pharmaceutical Ingredients.Affiliated research centres
- Learning Binding Affinities via Fine-tuning of Protein and Ligand Language Models
Accurate in-silico prediction of protein-ligand binding affinity is essential for efficient hit identification in large molecular libraries. Commonly used structure-based methods such as giga-docking often fail to rank compounds effectively, and free energy-based approaches, while accurate, are too computationally intensive for large-scale screening. Existing deep learning models struggle to generalize to new targets or drugs, and current evaluation methods do not reflect real-world performance accurately. We introduce BALM, a deep learning framework that predicts binding affinity using pretrained protein and ligand language models. BALM learns experimental binding affinities by optimizing cosine similarity in a shared embedding space. We also propose improved evaluation strategies with diverse data splits and metrics to better assess model performance. Using the BindingDB dataset, BALM shows strong generalization to unseen drugs, scaffolds, and targets. It excels in few-shot scenarios for targets such as USP7 and Mpro, outperforming traditional machine learning and docking methods, including AutoDock Vina. Adoption of the target-based evaluation methods proposed will allow for more stringent evaluation of machine learning-based scoring tools. Frameworks such as BALM show good performance, are computationally efficient, and are highly adaptable within this evaluation setting, making them practical tools for early-stage drug discovery screening.